Pandas cheat sheet¶¶
- Pandas Cheat Sheet
- Pandas Basics Cheat Sheet Printable
- Pandas Basics Cheat Sheet (2021) Python For Data Science
- Pandas Basics Cheat Sheet Free
- Panda Warmer Cheat Sheets
Pandas is Python Data Analysis library. Series and Dataframes are major data structures in Pandas. Pandas is built on top of NumPy arrays.
Python For Data Science Cheat Sheet Pandas Basics Learn Python for Data Science Interactively at www.DataCamp.com Pandas DataCamp Learn Python for Data Science Interactively Series DataFrame 4 Index 7-5 3 d c b A one-dimensional labeled array a capable of holding any data type Index Columns A two-dimensional labeled data structure with columns. Pandas library makes you feels very easy to manipulate your data. Here 1) the cheat sheet of Basic Pandas Python, and here 2) for the more advance data manipulation in Pandas (e.g., combine, join, concat, merge, etc). Import data in Python: How to import data to your python environment using pandas or numpy? Here the cheat sheet of importing.
ToC
- Series
- DataFrames
- Slicing and dicing DataFrames
- Conditional selection
- Operations on DataFrames
- DataFrame index
Pandas Cheat Sheet
Series¶¶
Series is 1 dimensional data structure. It is similar to numpy array, but each data point has a label in the place of an index.
Create a series¶¶
Thus Series can have different datatypes.
Operations on series¶¶
You can add, multiply and other numerical opertions on Series just like on numpy arrays.
When labels dont match, it puts a nan. Thus when two series are added, you may or may not get the same number of elements
DataFrames¶¶
Creating dataFrames¶¶
Pandas DataFrames are built on top of Series. It looks similar to a NumPy array, but has labels for both columns and rows.
reliability | cost | competition | halflife | |
---|---|---|---|---|
Car1 | 0.134302 | 0.625207 | 0.970981 | 0.717605 |
Car2 | 0.713766 | 0.773182 | 0.059689 | 0.450899 |
Car3 | 0.058990 | 0.904301 | 0.431487 | 0.087683 |
Car4 | 0.509891 | 0.501037 | 0.244279 | 0.763135 |
Slicing and dicing DataFrames¶¶
You can access DataFrames similar to Series and slice it similar to NumPy arrays
Access columns¶¶
Accessing using index number¶¶
If you don’t know the labels, but know the index like in an array, use iloc
and pass the index number.
Dicing DataFrames¶¶
Dicing using labels > use DataFrameObj.loc[[row_labels],[col_labels]]
cost | competition | |
---|---|---|
Car2 | 0.935368 | 0.719570 |
Car3 | 0.659950 | 0.605077 |
cost | competition | |
---|---|---|
Car2 | 0.935368 | 0.719570 |
Car3 | 0.659950 | 0.605077 |
Conditional selection¶¶
When running a condition on a DataFrame, you are returned a Bool dataframe.
reliability | cost | competition | halflife | |
---|---|---|---|---|
Car1 | 0.776415 | 0.435083 | 0.236151 | 0.169087 |
Car2 | 0.790403 | 0.987459 | 0.370570 | 0.734146 |
Car3 | 0.884783 | 0.233803 | 0.691639 | 0.725398 |
Car4 | 0.693038 | 0.716824 | 0.766937 | 0.490821 |
reliability | cost | competition | halflife | |
---|---|---|---|---|
Car3 | 0.884783 | 0.233803 | 0.691639 | 0.725398 |
Chaining conditions¶¶
In a Pythonic way, you can chain conditions
Multiple conditions¶¶
You can select dataframe elements with multiple conditions. Note cannot use Python and
, or
. Instead use &
, |
reliability | cost | competition | halflife | |
---|---|---|---|---|
Car1 | 0.776415 | 0.435083 | 0.236151 | 0.169087 |
Car2 | 0.790403 | 0.987459 | 0.370570 | 0.734146 |
Pandas Basics Cheat Sheet Printable
reliability | cost | competition | halflife | |
---|---|---|---|---|
Car1 | 0.776415 | 0.435083 | 0.236151 | 0.169087 |
Car2 | 0.790403 | 0.987459 | 0.370570 | 0.734146 |
Car3 | 0.884783 | 0.233803 | 0.691639 | 0.725398 |
Operations on DataFrames¶¶
Adding new columns¶¶
Create new columns just like adding a kvp to a dictionary.

reliability | cost | competition | halflife | full_life | |
---|---|---|---|---|---|
Car1 | 0.134302 | 0.625207 | 0.970981 | 0.717605 | 1.435210 |
Car2 | 0.713766 | 0.773182 | 0.059689 | 0.450899 | 0.901799 |
Car3 | 0.058990 | 0.904301 | 0.431487 | 0.087683 | 0.175366 |
Car4 | 0.509891 | 0.501037 | 0.244279 | 0.763135 | 1.526270 |
Dropping rows and columns¶¶
Row labels are axis = 0
and columns are axis = 1
reliability | cost | competition | halflife | |
---|---|---|---|---|
Car1 | 0.134302 | 0.625207 | 0.970981 | 0.717605 |
Car2 | 0.713766 | 0.773182 | 0.059689 | 0.450899 |
Car3 | 0.058990 | 0.904301 | 0.431487 | 0.087683 |
Car4 | 0.509891 | 0.501037 | 0.244279 | 0.763135 |
reliability | cost | competition | halflife | full_life | |
---|---|---|---|---|---|
Car1 | 0.134302 | 0.625207 | 0.970981 | 0.717605 | 1.435210 |
Car2 | 0.713766 | 0.773182 | 0.059689 | 0.450899 | 0.901799 |
Car4 | 0.509891 | 0.501037 | 0.244279 | 0.763135 | 1.526270 |
reliability | cost | competition | halflife | full_life | |
---|---|---|---|---|---|
Car1 | 0.134302 | 0.625207 | 0.970981 | 0.717605 | 1.43521 |
Car4 | 0.509891 | 0.501037 | 0.244279 | 0.763135 | 1.52627 |
DataFrame Index¶¶
So far, Car1
, Car2
.. is the index for rows. If you would like to set a different column as an index, use set_index
. If you want to make index as a column rather, and use numerals for index, use reset_index
Set index¶¶
reliability | cost | competition | halflife | car_names | |
---|---|---|---|---|---|
Car1 | 0.776415 | 0.435083 | 0.236151 | 0.169087 | altima |
Car2 | 0.790403 | 0.987459 | 0.370570 | 0.734146 | outback |
Car3 | 0.884783 | 0.233803 | 0.691639 | 0.725398 | taurus |
Car4 | 0.693038 | 0.716824 | 0.766937 | 0.490821 | mustang |
reliability | cost | competition | halflife | car_names | |
---|---|---|---|---|---|
car_names | |||||
altima | 0.776415 | 0.435083 | 0.236151 | 0.169087 | altima |
outback | 0.790403 | 0.987459 | 0.370570 | 0.734146 | outback |
taurus | 0.884783 | 0.233803 | 0.691639 | 0.725398 | taurus |
mustang | 0.693038 | 0.716824 | 0.766937 | 0.490821 | mustang |
index | reliability | cost | competition | halflife | car_names | |
---|---|---|---|---|---|---|
0 | Car1 | 0.776415 | 0.435083 | 0.236151 | 0.169087 | altima |
1 | Car2 | 0.790403 | 0.987459 | 0.370570 | 0.734146 | outback |
2 | Car3 | 0.884783 | 0.233803 | 0.691639 | 0.725398 | taurus |
3 | Car4 | 0.693038 | 0.716824 | 0.766937 | 0.490821 | mustang |
This cheat sheet is a quick reference for data wrangling with Pandas, complete with code samples.
by Karlijn Willems
Pandas Basics Cheat Sheet (2021) Python For Data Science
By now, you’ll already know the Pandas library is one of the most preferred tools for data manipulation and analysis, and you’ll have explored the fast, flexible, and expressive Pandas data structures, maybe with the help of DataCamp’s Pandas Basics cheat sheet.
Yet, there is still much functionality that is built into this package to explore, especially when you get hands-on with the data: you’ll need to reshape or rearrange your data, iterate over DataFrames, visualize your data, and much more. And this might be even more difficult than “just” mastering the basics.
That’s why today’s post introduces a new, more advanced Pandas cheat sheet.
It’s a quick guide through the functionalities that Pandas can offer you when you get into more advanced data wrangling with Python.
(Do you want to learn more? Start our Pandas Foundations course for free now or try out our Pandas DataFrame tutorial! )
The Pandas cheat sheet will guide you through some more advanced indexing techniques, DataFrame iteration, handling missing values or duplicate data, grouping and combining data, data functionality, and data visualization.
Pandas Basics Cheat Sheet Free
In short, everything that you need to complete your data manipulation with Python!
Panda Warmer Cheat Sheets
Don’t miss out on our other cheat sheets for data science that cover Matplotlib, SciPy, Numpy, and the Python basics.
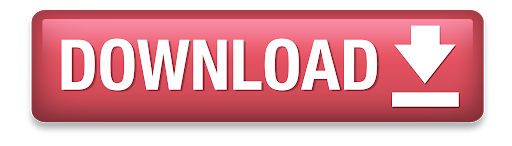